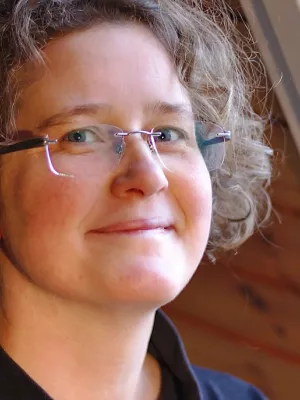
Ullrika Sahlin
Universitetslektor
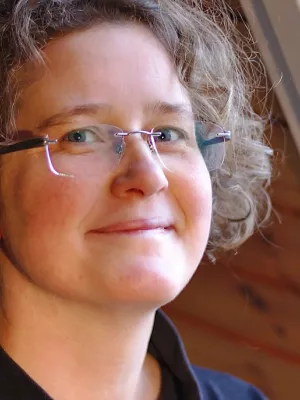
Iterative importance sampling with Markov chain Monte Carlo sampling in robust Bayesian analysis
Författare
Summary, in English
Bayesian inference under a set of priors, called robust Bayesian analysis, allows for estimation of parameters within a model and quantification of epistemic uncertainty in quantities of interest by bounded (or imprecise) probability. Iterative importance sampling can be used to estimate bounds on the quantity of interest by optimizing over the set of priors. A method for iterative importance sampling when the robust Bayesian inference relies on Markov chain Monte Carlo (MCMC) sampling is proposed. To accommodate the MCMC sampling in iterative importance sampling, a new expression for the effective sample size of the importance sampling is derived, which accounts for the correlation in the MCMC samples. To illustrate the proposed method for robust Bayesian analysis, iterative importance sampling with MCMC sampling is applied to estimate the lower bound of the overall effect in a previously published meta-analysis with a random effects model. The performance of the method compared to a grid search method and under different degrees of prior-data conflict is also explored.
Avdelning/ar
- Centrum för miljö- och klimatvetenskap (CEC)
- eSSENCE: The e-Science Collaboration
- MERGE: ModElling the Regional and Global Earth system
- Matematisk statistik
- Beräkningsvetenskap för hälsa och miljö
Publiceringsår
2022-12
Språk
Engelska
Publikation/Tidskrift/Serie
Computational Statistics and Data Analysis
Volym
176
Dokumenttyp
Artikel i tidskrift
Förlag
Elsevier
Ämne
- Probability Theory and Statistics
Nyckelord
- Bounds on probability
- Effective sample size
- Meta-analysis
- Random effects model
- Uncertainty quantification
Status
Published
Forskningsgrupp
- Computational Science for Health and Environment
ISBN/ISSN/Övrigt
- ISSN: 0167-9473