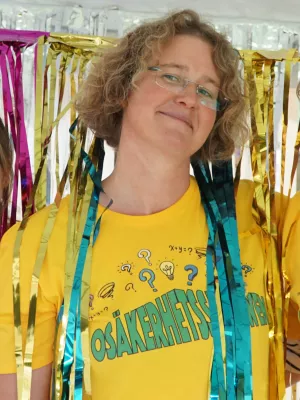
Ullrika Sahlin
Senior lecturer
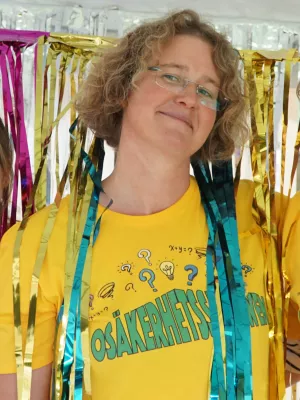
Hybrid elicitation and quantile-parametrized likelihood
Author
Summary, in English
This paper extends the application of quantile-based Bayesian inference to probability distributions defined in terms of quantiles of observable quantities. Quantile-parameterized distributions are characterized by high shape flexibility and parameter interpretability, making them useful for eliciting information about observables. To encode uncertainty in the quantiles elicited from experts, we propose a Bayesian model based on the metalog distribution and a variant of the Dirichlet prior. We discuss the resulting hybrid expert elicitation protocol, which aims to characterize uncertainty in parameters by asking questions about observable quantities. We also compare and contrast this approach with parametric and predictive elicitation methods.
Department/s
- Centre for Environmental and Climate Science (CEC)
- BECC: Biodiversity and Ecosystem services in a Changing Climate
- Computational Science for Health and Environment
- MERGE: ModElling the Regional and Global Earth system
Publishing year
2024-02
Language
English
Publication/Series
Statistics and Computing
Volume
34
Document type
Journal article
Publisher
Springer
Topic
- Probability Theory and Statistics
Keywords
- Bayesian analysis
- Expert knowledge elicitation
- Indirect inference
- Quantile-based distributions
- Quantile-parameterized distributions
Status
Published
Research group
- Computational Science for Health and Environment
ISBN/ISSN/Other
- ISSN: 0960-3174